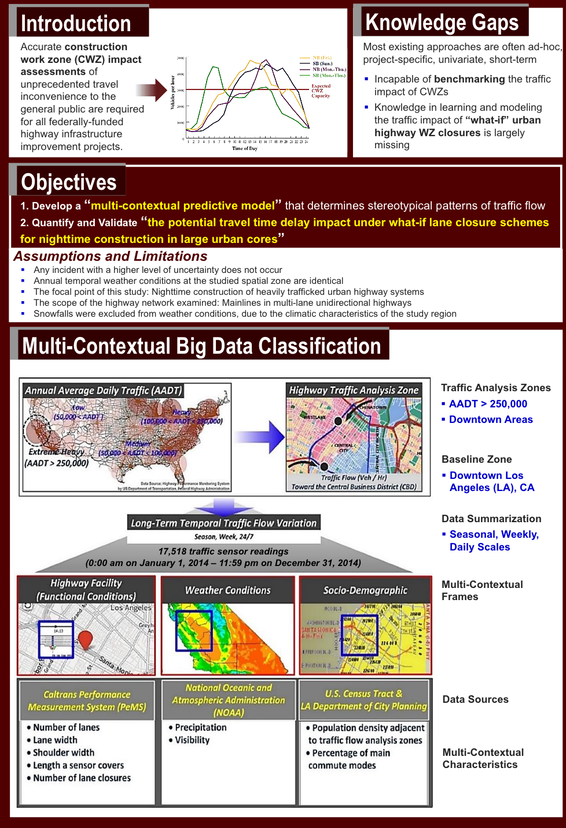
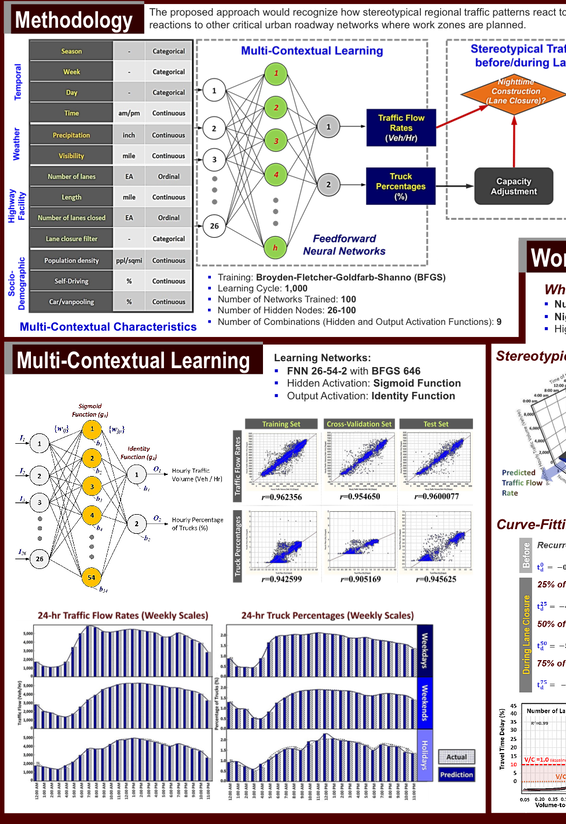
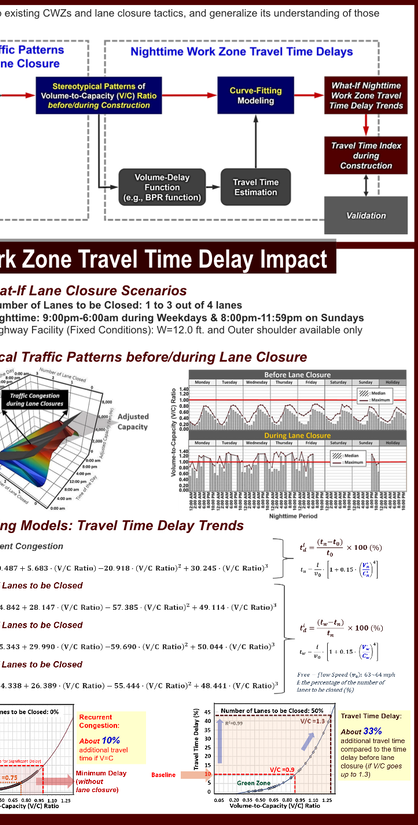
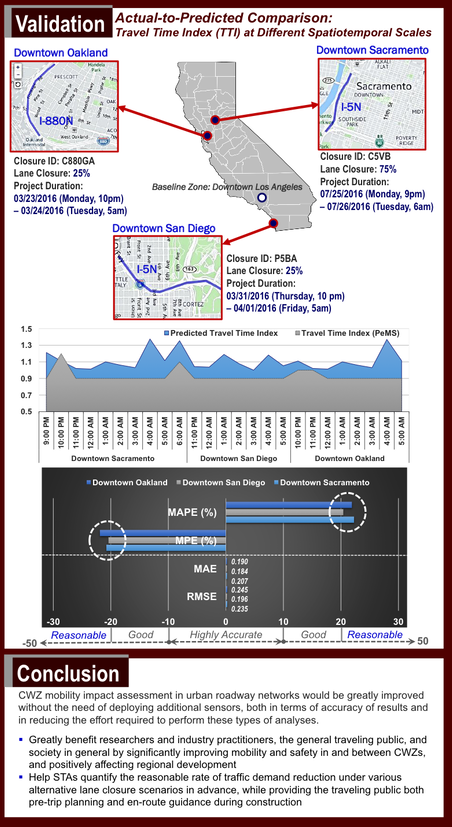
SRP1: AI-Powered Mobility Impact Assessment of Highway Construction Alternatives
This project aims to improve mobility and safety in and around highway construction work zones (CWZs) by creating and testing a focused cyber-physical decision-support system. This system would predict potential work zone impact on motorists, affected communities, and businesses. To achieve this goal, this research investigates an integrative high-fidelity computing concept that blends novel deep-learning traffic pattern prediction algorithms based on inputs from existing sensors located on roadway segments in an urban corridor with CWZ impact assessment techniques. We propose a decision-support model: Spatiotemporal Work Zone Assessment for Transportation Management Plans (SWAT).
Utilizing deep-learning methods, SWAT will automatically recognize how stereotypical regional traffic patterns react to existing CWZs and implement transportation management plan (TMP) strategies based on roadway characteristics as well as socioeconomic inputs. Then, SWAT will automatically generalize its understanding of those reactions to other roadway networks where work zones are planned. In this way, CWZ impact assessment in urban roadway networks would be greatly improved, both in terms of accuracy of results and in reducing the effort required to perform these types of analyses: the image below summarizes the outcomes of a study specifically aimed at developing an AI predictive model to determine the mobility impact of various nighttime lane closure scenarios on an urban highway network in Southern California.